Through my involvement in arsenic research, I heard about the following news item (see below). It's an interesting cost-benefit case, especially since lots of people drink bottled water even though their local water supply is completely safe. I think I would prefer that my town put in the effort to have lower arsenic, but perhaps I'm just too attuned to the arsenic problem because I work in that area. But my favorite part of the article is that Dickensian name, Benjamin H. Grumbles.
Results matching “R”
Bob Erikson, Chris Wlezien and I have been working on a paper about midterm balancing. We see it as still very viable and find solid evidence that it happens. In what follows, I have copied in portions of the paper. They will reveal what we did, what we found and what it all means. The paper is under review at POQ.
In 1998, as the unpopular impeachment of President Bill Clinton was unfolding, Clinton’s Democrats gained seats in the House of Representatives. In 2002, in the shadow of 9/11, President George W. Bush’s Republicans gained House seats as well. These two recent instances might make it seem commonplace for the presidential party to gain House seats at midterm. Indeed, the early interpretations of 2002 by Jacobson (2003) and Campbell (2003) emphasized the theme that this election unfolded as normal politics. Jacobson even chose not to remark about the historical significance of the presidential party gaining seats. The historical pattern, of course, is that the presidential party loses seats at midterm. This in fact had been more than simply a pattern, and almost a deterministic law of politics. From 1842 through 1994, the presidential party gained seats (as a proportion of the total) only once—in 1934 as the FDR-led Democrats’ surged with a gain of nine seats. This was a spectacular run of 38 presidential party losses in 39 midterm elections. Clearly, forces are at work in American politics to diminish the electoral standing of the presidential party at midterm.
Mike Shores writes,
I am a junior at Roslyn High School (Roslyn, NY). I am currently enrolled in my schools Independent Social Science Research Program, where I am studying the impact of teachers' political biases on essay grading. However, I have run into some statistical problems and am wondering if you would be able to help me.In my experiment there are two independent variables. Teachers were assigned to read one of two essays (liberal or conservative) and to report their personal political orientation (liberal or conservative) on a 6-point scale. I was interested in how these two factors affected the grade teachers assigned the essay.
I initially ran a two-way ANOVA and to do so I defined participants as liberal if rated themselves from 1 to 3 or conservative if they rated themselves as a 4 to 6. In doing so, however, I wonder if I’m giving up some statistical power and so I am trying to figure out if there is a better way to analyze the data.
Another possibility I thought of was to analyze the data from the liberal and conservative essays separately and run correlations between their political beliefs and the grade they gave the essay. I was wondering if you had any suggestions about how I should proceed.
My reply:
First of all, I'm impressed that you got the teachers to agree to grade extra papers for you!
My quick answer is that you'd be better off calculating averages and standard errors, and making scatterplots, rather than doing anova. Probably the best thing would be 2 scatterplots, one for the conservative essays and one for the liberal essays, plotting teacher's self-evalutation vs. grade given to the paper. You can then compute the correlation for each of the scatterplots (and you can check if each of these is statistically significantly different from 0).
Or you can run a regression, predicting the grade of the essay given 3
variables:
x1 = the teachers' poliical orientation
x2 = a variable that is +1 if the essay is conservative and -1 if liberal
x3 = an interaction of x1 and x2 (i.e., x1*x2)
There are probably other good ways to analyze the data too. But, yeah, you shouldn't simplify the 1-6 scale to a binary scale. that's just throwing away information.
If anybody has other thoughts, feel free to pass them along.
I wonder if Seth has considered performing rat experiments on his diet? I remember Seth telling me that rat experiments are pretty cheap (i.e., you can do them without getting external grant funding), and he discusses in his book how much he's learned from earlier rat experiments.
Marty Ringo send me the following comments on my paper on the (mis)application of the Prisoner's Dilemma to trench warfare. I appreciate the comments, especially given that the closest I've come to military service was the Boy Scouts when I was 11, and the last time I was in combat was a fistfight in 7th grade. Anyway, Ringo writes,
As an ex-NCO (non-commissioned officer, i.e. sergeant) I have varying degrees of prejudice about academics writing about combat. This is, of course, self-contradicting since I am a semi-academic and most of what I know about combat has been acquired from reading in my post-military life.S.L.A. Marshall's famous study on combat fire found that few, maybe something like 20%, of the soldiers in WW II actually fired their weapons in combat. Marshall's research methods have been since questioned, but the point he raised still lingers. A famous WW I joke pertains to this issue.
Gregor writes in with a (long) question. I'll give the question and then my reply for each.
Gregor's first question:
Gregor writes,
I would like to hear your opinion on Paul Johnson comments here, where this link is provided.
Larry Bartels spoke in our seminar the other day and talked about this paper on Democrats, Republicans, and the economy. It started with this graph, which showed that incomes have grown faster under Democratic presidents, especially on the low end of the scale:
He looked at it in a number of ways, and the evidence seemed convincing that, at least in the short term, the Democrats were better than Republicans for the economy. This is consistent with Democrats' general policies of lowering unemployment, as compared to Republicans lowering inflation, and, by comparing first-term to second-term presidents, he found that the result couldn't simply be explained as a rebound or alternation pattern.
But then, he asked, why have the Republicans won so many elections? Why aren't the Democrats consistently dominating? Non-economic issues are part of the story, of course, but lots of evidence shows the economy to be a key concern for voters, so it's still hard to see how, with a pattern such as shown above, the Republicans could keep winning.
Larry had two explanations.
Seth Roberts's book, The Shangri-La Diet: The No Hunger Eat Anything Weight-Loss Plan, is out.. Maybe I can be the first person to review it. (I've known Seth for over 10 years; we co-taught a class at Berkeley on left-handedness.)
Seth figured out his basic idea--that drinking unflavored sugar water lowers his "setpoint," thus making it easy for him to lose weight--about 10 years ago, following several years of self-experimentation (see here for a link to his article). Since then, he's tried it on other people, apparently with much success, and generalized it to inclde driking unflavored oil as a different option for keeping the setpoint down every day.
The book itself describes the method, and the theory and experimental evidence behind it. It seems pretty convincing to me, although I confess I haven't tried the diet myself. I suppose that thousands will, now that the book has come out. If it really is widely successful, I'll just have to say that I'm impressed with Seth for following this fairly lonely research path for so many years. I had encouraged him to try to study the diet using a controlled experiment, but who knows, maybe this is a better approach, The unflavored-oil option seems to be a good addition, in making the diet less risky for diabetics.
Some other random notes:
1. I like the idea of a moving setpoint. Although then maybe the word "setpoint" is inappropriate?
2. The book is surprisingly readable, given that I already knew the punchline (the diet itelf). A bit like the book "Dr. Jekyll and Mr. Hyde," which is actually suspenseful, even though you know from the beginning that they're the same guy.
3. In the appendix, Seth describes some published research that influenced his work. The researchers were from fairly obscure places--Laval University, Brooklyn College, and Monell Chemical Sciences Institute. Perhaps this is because animal nutrition research is an obscure field that flourishes in out-of-the-way places? Or perhaps because there are literally millions of scientific researchers around the world, and it's somewhat random who ends up at the "top" places?
4. Near the end of the book, Seth discusses ways in which the food industry could profit from his dieting insights and make money offering foods that lower the setpoint. That's a cool idea--to try to harness these powerful forces in society to move in that direction.
5. With thousands of people trying this diet, will there be a way to monitor its success? Or maybe now, some enterprising researchers will do a controlled experiment. It really shouldn't be difficult at all to do such a study; perhaps it could be a good project for some class in statistics or psychology or nutrition.
More
P.S. See Alex's blurb here, which I guess a few thousand more people will notice. I'm curious what Alex (and others) think about my point 5 above. In a way, you could say it's a non-issue, since each individual person can see if the diet works for him or her. But for scientific understanding, if nothing else, I think it would be interesting to learn the overall effectiveness (or ineffectiveness) of the diet.
P.P.S. Regarding point 1 above, Denis Cote writes,
Indeed, there is some talk about a settling point which is a more appropriate label. (see Pinel et al 2000. Hunger, Eating, and Ill Health, American Psychologist. 55(10), 1105-1116.
I'll have to take a look. The American Psychologist is my favorite scientific journal in the sense of being enjoyable and interesting to read.
From Freakonomics, a link to this paper by David Cutler and Edward Glaeser. Here's the abstract:
While Americans are less healthy than Europeans along some dimensions (like obesity), Americans are significantly less likely to smoke than their European counterparts. This difference emerged in the 1970s and it is biggest among the most educated. The puzzle becomes larger once we account for cigarette prices and anti-smoking regulations, which are both higher in Europe. There is a nonmonotonic relationship between smoking and income; among richer countries and people, higher incomes are associated with less smoking. This can account for about one-fifth of the U.S./Europe difference. Almost one-half of the smoking difference appears to be the result of differences in beliefs about the health effects of smoking; Europeans are generally less likely to think that cigarette smoking is harmful.
This is an interesting problem, partly because evidence suggests that anti-smoking programs are ineffective, but there is a lot of geographic variation in smoking rates across countries, as well as among U.S. states (and among states in India too, as S. V. Subramanian has discussed). It's encouraging to think that better education could reduce smoking rates.
Amazingly, "in Germany only 73 percent of respondents said that they believed that smoking causes cancer." Even among nonsmokers, only 84% believed smoking caused cancer! I'd be interested in knowing more about these other 16%. (I guess I could go to the Eurobarometer survey and find out who they are.)
?
There are a couple things in the paper I don't quite follow. On page 13, they write, "there is a negative 38 percent correlation coefficient between this regulation index and the share of smokers in a state. A statistically significant negative relationship result persists even when we control for a wide range of other controls including tobacco prices and income. As such, it is at least possible that greater regulation of smoking in the U.S. might be a cause of the lower smoking rate in America." But shouldn't they control for past smoking rates? I imagine the regulations are relatively recent, so I'd think it would be appropriate to compare states that are comparable in past smoking rates but differ in regulations.
Finally, there's some speculation at the end of the paper about how it is that Americans have become better informed than Europeans about the health effects of smoking. They write, "while greater U.S. entrepreneurship and economic openness led to more smoking during an earlier era (and still leads to more obesity today), it also led to faster changes in beliefs about smoking and ultimately less cigarette consumption." I don't really see where "entrepreneurship" comes in. I had always been led to believe that a major cause of the rise in smoking in the U.S. in the middle part of the century was that that the GI's got free smokes in their rations. For anti-smoking, I had the impression that federalism had something to do with it--the states got the cigarette taxes, and the federal government was free to do anti-smoking programs. But I'm not really familiar with this history so maybe there's something I'm missing here.
P.S. Obligatory graphics comments:
Junk Charts features these examples of pretty but not-particularly-informative bubble plots from the New York Times. If you like those, you'll love this beautiful specimen that Jouni sent me awhile ago. As Junk Charts puts it, "perhaps the only way to read their intention is to see them as decorated data tables, in other words, as objets d'art rather than data displays."
I'll have to say, though, the bubble charts do display qualitative comparisons better than tables do. But, yeah, "real" graphs (dot plots, line plots, etc) would be better.
Shang Ha writes,
I have a quick question on Bayesian hierarchical models.I've never gotten a solid answer about the issue of how many respondents should be nested within level-2 units. If I can remember right, Bryk and Raudenbush suggest AT LEAST THREE, but recommend more for conventional HLM. But I cannot find any suggestions in terms of Bayesian hierachical models.
The survey dataset I have comprises the following:
68 metropolitan areas (the number of respondents ranges from 5 to 37)
187 zip code areas (the # of respondents ranges from 1 to 13)
211 census tracts (the # of respondents ranges from 1 to 10)Can I build hierarchical models using zip code areas and census tracts? If it is not possible in the case of
conventional HLM, then can I apply Bayesian hierarchical model?
My response: yes, you can do it, using either Bayesian or non-Bayesian hierarchical modeling. The two varieties of hierarchical modeling are almost the same; the main difference is that the Bayesian models the variance parameters and the non-Bayesian uses point estimates. Typically there is no real difference between the Bayesian and non-Bayesian unless there are variance parameters estimated near zero or group-level correlation parameters estimated near 1 or -1. All hierarchical models are Bayesian for the purpose of estimating the varying coefficients.
You don't need 3 observations per group to estimate a multilevel model. 2 observations per group is fine. In fact, it's even ok to have some groups with 2 observations and some with only 1 observation. I don't know why anyone would say that 3 or more observations per group are required.
I'm not much of a sports fan, but I enjoy reading "King Kaufman's Sports Daily" at Salon.com. (I think Kaufman's column may be only available to "Premium" (paid) subscribers). For the past few years, Kaufman has tracked the performance of self-styled sports "experts" in predicting the outcome of the National Collegiate Athletic Association's (NCAA) men's basketball tournament, which begins with 64 teams that are selected by committee and supposedly represent (more or less) the best teams in the country, and ends with a single champion via a single-elimination format. Many people wager on the outcome of the tournament --- not just who will become champion, but the entire set of game outcomes --- by entering their predictions into a "pool" from which the winner is rewarded.
Last year and this year, Kaufman included the "predictions" of his son Buster (now three years old). Last year Buster flipped a coin for every outcome, and did not perform well; this year, he followed a modified strategy that is essentially a way of sampling from a prior distribution derived from the official team rankings created by the NCAA selection committee.
The Pool o' Experts features a roster of national typists and chatterers, plus you, the unwashed hordes as represented by the CBS.SportsLine.com users' bracket, and my son, Buster, the coin-flippinest 3-year-old in the Milky Way. [Kaufmann later explains "Buster's coin-flipping strategy was modified again this year. Essentially, he picked all huge favorites, flipped toss-up games, and needed to flip tails twice to pick the upsets in between. Write me for details if this interests you, but think really hard before you do that, and maybe call your therapist."]
To answer the inevitable question: Yes, Buster really exists. When football season comes in five months and he's still 3, I'll get letters saying it seems like he's been 3 for about two years, which says something about how we perceive the inexorable crawl of time. But I don't know what.
Anyway, correct predictions earn 10 points in the first round, 20 in the second, and 40, 80, 120 and 160 for subsequent rounds.
Someone who goes by the name "mr_unix" writes:
In your blog entry, there is reference to a public document called "Reference Manual on Scientific Evidence." I downloaded it and tried to read the chapter on multiple regression by Rubinfeld. I couldn't read it because the footnotes were so copious and interfering. I removed them with awk and reformatted the chapter to resemble an ordinary (!) monograph. Some people will benefit from it, I think. It's attached in MS Word format.
Here it is. I don't agree with everything in it, but it's basically reasonable.
James Stamey writes,
I thought about some things I have seen on your blog as I was reading through another website I keep up with, getreligion.org. (The website is run by several media members who happen to be religious with the premise that in general the media does not "get" religion.) Anyway, the red/blue state ideas come up all the time and recently the conversation was about people who economically benifit from democrat policies voting republican and people who economically would benifit from republican policies, voting democrat. A couple of the comments were:I am one of those people who generally fares better economically under Democrats, but generally votes Republican due to, yes, abortion. While I have certainly voted for Dems, even pro-choice Dems, under specific circumstances, the murder of unborn children trumps my bank account in the grand scheme of things.and
I’m one of those people would generally fares better economically under Republicans, but my wife and I vote for Democrats because of social issues. . . . If you are concerned about poverty, the death penalty, just war, a foreign policy based on human rights, a humane immigration policy, and policies which promote toleance and diversity, we put our economic needs aside and vote for Democrats.
My reply: I have two thoughts on this. First, I find it interesting that these people associate economic voting with the idea of voting for personal gain. Theory and data both suggest that people vote for what they think is good for the country economically, not necessarily what they think is good for them. I'd be curious what opinions the people quoted above would have about national economic policies.
This item from Carrie's blog reminds me of a trick I used to do when giving seminars: I'd give out approximately 2/3 as many handouts as there were people in the audience. That way they'd have to share the handouts, and they'd value them more. When I would give enough handouts for everybody, people would just put them in their notebook and not bother looking at them.
I have a friend --- a prominent statistician --- who doesn't like wine but drinks a glass a day because it is (supposedly) good for his heart. But an article in the San Francisco Chronicle reports on a new study that says that all 50 or so of the published articles that found a benefit are performing an analysis with the same serious flaw:
With all the contradictory claims made these days about the health benefits of low-fat diets, the harm of hormone replacements and the dangers of pain relievers, at least we still know that a drink or two a day is good for the heart.
Well, maybe not.
Researchers at UCSF pored through more than 30 years of studies that seem to show health benefits from moderate alcohol consumption, and concluded in a report released today that nearly all contained a fundamental error that skewed the results.
That error may have led to an erroneous conclusion that moderate drinkers were healthier than lifelong abstainers. Typically, studies suggest that abstainers run a 25 percent higher risk of coronary heart disease.
Without the error, the analyses shows, the health outcomes for moderate drinkers and non-drinkers were about the same.
"This reopens the debate about the validity of the findings of a protective effect for moderate drinkers, and it suggests that studies in the future be better designed to take this potential error into account,'' said Kaye Fillmore, a sociologist at the UCSF School of Nursing and lead author of the study.
The common error was to lump into the group of "abstainers" people who were once drinkers but had quit.
Many former drinkers are people who stopped consuming alcohol because of advancing age or poor health. Including them in the "abstainer" group made the entire category of non-drinkers seem less healthy in comparison.
This type of error in alcohol studies was first spotted by British researcher Dr. Gerry Shaper in 1988, but the new analysis appears to show that the problem has persisted.
Fillmore and colleagues from the University of Victoria, British Columbia; and Curtin University, in Perth, Australia, analyzed 54 different studies examining the relationship between light to moderate drinking and health. Of these, only seven did not inappropriately mingle former drinkers and abstainers.
All seven of those studies found no significant differences in the health of those who drank -- or previously drank -- and those who never touched the stuff. The remaining 47 studies represent the body of research that has led to a general scientific consensus that moderate drinking has a health benefit.
[article continues]
The article does go on to say that some researchers had looked at this effect previously, that there is still evidence (like cholesterol levels) that moderate drinking is good, yada yada. But still, this does look like a serious flaw. And it also seems "obvious." Is it?
I got an email from a friend who used to live in New York and now lives in Seattle:
Andrew Sutter writes,
The Serenity Prayer, attributed to Reinhold Niebuhr and now associated with Alcoholics Anonymous, goes,
God give me the serenity to accept things which cannot be changed; Give me courage to change things which must be changed; And the wisdom to distinguish one from the other.
I think this would make a great classroom example of Venn diagrams (as used for set theory and probability). There are two sets:
A: "things which cannot be changed"
B: "things which must be changed"
and the prayer implicitly assumes that A and B are disjoint and exhaustive (that is, that every item in the universe of "things" being considered is either in A or in B, but not both).
But if you draw the Venn diagram, you can see the possibility of:
not-A and not-B: this is ok, things which can be changed but do not need to be changed
A and B: this is bad, these are the things which cannot be changed but must be changed!
This is a great example, in that the rhetoric of the prayer is so compelling that it's easy to miss, at first, these other two categories, but the Venn diagram makes it clear. Also, many students will already have been exposed to this prayer, and the others will probably find it interesting. How does the Venn diagram version affect how the prayer says we should live our lives?
P.S. The above version of the prayer is from Niebuhr. As Jim Lebeau notes in a comment, the actual version used by AA goes "God grant us the serenity to accept the things we cannot change, courage to change the things we can . . .", which doesn't quite work as a Venn diagram example.
P.P.S. The pretty picture below is from Will Fitzgerald (see comments).
Dan Goldstein set up this demo you can show to your students to understand least squares. It's pretty cool, although there's something about those square boxes that I find disconcerting. I don't usually think of the squaring in least squares as actual sizes of physical squares. So I'm thinking that, for some students, the square boxes will be a help; for others, a distraction. I'd also like to see more than 4 points, and I'd like to drag and manipulate the points and the line themselves, rather than simply clicking on buttons to move the line around. But now that this has been set up, presumably it won't be hard to add these improvements if appropriate.
Newcomb's paradox is considered to be a big deal, but it's actually straightforward from a statistical perspective. The paradox goes as follows: you are shown two boxes, A and B. Box A contains either $1 million or $0, and Box B contains $1000. You are given the following options: (1) take the money (if any) that's in Box A, or (2) take all the money (if any) that's in Box A, plus the $1000 in Box B. Nothing can happen to the boxes between the time that you make the decision and when you open them and take the money, so it's pretty clear that the right choice is to take both boxes. (Well, assuming that an extra $1000 will always make you happier...)
The hitch is that, ahead of time, somebody decided whether to put $1 million or $0 into Box A, and that Somebody did so in a crafty way, putting in $1 million if he or she thought you would pick Box A only, and $0 if he or she thought you would pick Box A and B. Let's suppose that this Somebody is an accurate forecaster of which option you would choose. In that case, it's easy to calculate that the expected gain of people who pick only Box A is greater than the expected gain of people who would pick both A and B. (For example, if Somebody gets it right 70% of the time, for either category of person, then the expected monetary value for the "believers" who pick only box A is 0.7*($1,000,000) + 0.3*0 = $700,000, and the expected monetary value for the "greedy people" who pick both A and B is 0.7*$1000 + 0.3*$1,001,000 = $301,000.) So the A-pickers do better, on average, than the A-and-B-pickers.
The paradox
The paradox, as has been stated, is that from the perspective of the particular decision, it's better to pick A and B, but from the perspective of expected monetary value, it appears better to pick just A.
Resolution of the paradox
It's better to pick A and B. The people who pick A do better than the people who pick A and B, but that doesn't mean it's better for you to pick A. This can be explained in a number of statistical frameworks:
- Ecological correlation: the above expected monetary value calculation compares the population of A-pickers with the population of A-and-B-pickers. It does not compare what would happen to an individual. Here's an analogy: one year, I looked at the correlation between students' midterm exam scores and the number of pages in their exam solutions. There was a negative correlation: the students who wrote the exams in 2 pages did the best, the students who needed 3 pages did a little worse, and so forth. But for any given student, writing more pages could only help. Writing fewer pages would give them an attribute of the good students, but it wouldn't actually help their grades.
- Random variables: label X as the variable for whether the Somebody would predict you are an A-picker, and label Y as the decision you actually take. In the population, there is a positive correlation between X and Y. But X occurs before Y. Changing Y won't change X, any more than painting dots on your face will give you chicken pox. Yes, it would be great to be identified as an A-picker, but picking A won't change your status on this.
One more thing
Some people have claimed to "resolve" Newcomb's paradox by saying that this accurate-forecasting Somebody can't exist; the Somebody is identified with God, time travel, reverse causation, or whatever. But from a statistical point of view, it shouldn't be hard at all to come up with an accurate forecast. Just do a little survey, ask people some background questions (age, sex, education, occupation, etc.), then ask them if they'd pick A or A-and-B in this setting. Even a small survey should allow you to fit a regression model that would predict the choice pretty well. Of course, you don't really know what people would do when presented with the actual million dollars, but I think you'd be able to forecast to an accuracy of quite a bit better than 50%, just based on some readily-available predictors.
It is not unusual for statisticians to check their model with cross-validation. Last week we have seen that several replications of cross-validation reduce the standard error of mean error estimate, and that cross-validation can also be used to obtain pseudo-posteriors alike those using Bayesian statistics and bootstrap. This posting will demonstrate that the choice of prior depends on the parameters of cross-validation: a "wrong" prior may result in overfitting or underfitting according to the CV criterion. Furthermore, the number of folds in cross-validation affects the choice of the prior.
From June 17-24, UCLA will again host its Undergraduate Program in Statistics. A flyer for the program is available here. This week-long event is designed to introduce students to the
exciting challenges facing today's "data scientists. For more information or for an application, please visit this web site.
A colleague and ran into a problem for which we felt compelled to fit a sophisticated model, or at least a complicated one, just to perform an exploratory data analysis.
The data are measurements of the "leakiness" of various commercial buildings: if you pressurize them to a particular level compared to the outdoors, how much air escapes? Enormous fans are used to pressurize the building, and the amount of flow that the fans provide in order to attain a given pressure drop is recorded. Data come from various countries (US, SWEDen, FRAnce, etc.), various categories of buildings (EDUcational, OFFice, WAREhouse, etc.), and various construction types (MASonry, METal frame, TILT-up, etc.) We also know how tall the building is (in number of floors), and we know the approximate building footprint, and sometimes a few other things like the age of the building.
This figure shows the log (base 10) of the leakiness, in liters per second per square meter of building shell, at a 50 Pascal pressure differential, for each building, grouped by country/category/construction, and using different digits to indicate the height of the building (1 = single story, 2 = 2-3 stories, 3 = 4-5 stories, and 4 = 6 or more stories).
Gregor Gorjanc writes,
A few years ago I went to a Sunday afternoon party in Brooklyn at the home of a couple who had 2 kids. One was a 7-year-old boy who, I'd been told, loved to play chess--he was a great chess player, but didn't get much of a chance to play (his parents barely knew the rules). When I was introduced to him I told him I'd like to play chess--he was very happy and we set up the board. Right before we started, he said to me, "I'm gonna kick your ass!" I tried to gently explain to him that he couldn't really kick my ass at chess, he's just 7 years old and I'm an adult, etc. (I'm not a particularly good chess player. But I know I can beat a 7-year-old kid.) He didn't believe me, kept insisting he would kick my ass. I quickly conferred with his mother and asked whether it was OK for me to beat him and she said, yeah, it would actually be good for him to lose a little. So we played, I tried to play like a kid, chasing his pieces around. The game was OK, he was actually pretty good, but I followed his mom's instructions and beat him. We played again with similar results. He was fine with it.
Anyway, the point of this story is not that I have the amazing skills necessary to beat a little kid in chess--I just think it's funny that so few adults in that corner of Brooklyn know how to play chess. They have chess in the schools, and somehow this kid picked up the notion that kids know how to play chess but adults don't.
Simon Urbanek from AT&T Labs gave an excellent presentation last week in our statistical graphics class, on the topic of interactive graphics. He did some demos, and here are his slides. I think there's the potential for more work in this area, especially in graphical summaries of fitted models.
P.S. Thanks to Antony Unwin for recommending that we invite Simon to speak.
Aleks Jakulin gave a cool talk on color graphics for our seminar. Here are the slides.
Although this isn't really news anymore, Washington Post writes that Democrats' Data Mining Stirs an Intraparty Battle. The big plan is that the Democrat party will build a database of all its voters, as to be able to send customized messages and identify new potential voters, but hopefully also listen to opinions in a more effective manner and obtain feedback through detecting party swings.
I have come across an excellent page on R Graphics, the R Graph Gallery. There are a few innovative visualizations, and the page demonstrates how a vibrant internet community can create a comprehensive dictionary of visualizations. Each graph comes along with the source.
Just as an example, here are two graphs with best scores (the images are clickable):
(similar to our visualizations of the US Senate)
A Bayesian posterior distribution of a parameter demonstrates the uncertainty that arises from being a priori uncertain about the parameter values. But in a classical setting, we can obtain a similar distribution of parameter estimates through the uncertainty in the choice of the sample. In particular, using methods such as bootstrap, cross-validation and data splitting, we can perform the simulation. It is then interesting to compare the Bayesian posteriors with these. Finally, we can also compare the classical asymptotic distributions of parameters (or functionals of these parameters) with all of these.
Let us assume the following contingency table from 1991 GSS:
Belief in Afterlife | ||
Gender | Yes | No/Undecided |
Females | 435 | 147 |
Males | 375 | 134 |
We can summarize the dependence between gender and belief with an odds ratio. In this case, the odds ratio is simply θ= (435*134)/(147*375)=1.057 - very close to independence. It is usually preferable to work with the natural logarithm of the odds ratio, as its "distribution" is less skewed. Let us now examine the different distributions of log odds ratio (logOR) for this case, using bootstrap, cross-validation, Bayesian statistics and asymptotics.
In Bayesian statistics one often has to justify the prior used. While picking the prior may be seen as a subjective preference, if a different person disagrees with the prior, the conclusions would not be acceptable. For the results of the analysis to be acceptable, a large number of people would need to agree with the prior. Priors are not particularly easy to understand and evaluate, especially when the models become complex.
On the other hand, the fundamental idea of data splitting is very intuitive: we hide one part of the data, learn on the rest, and then check our "knowledge" on what was hidden. There is a hidden parameter here: how much we hide and how much we show. Traditionally, "cross-validation" meant the same as is currently meant by "leave-one-out": we hide a single case, model from all the others, and then compute the error of the model on that hidden case.
As to remove the influence of a particular choice of what to show and what to hide, we ideally average over all show/hide partitions of the data. The contemporary understanding of "k-fold cross-validation" is different from the traditional one. We split the cases at random into k groups, so that each group has approximately equal size. We then build k models, each time omitting one of the groups. We evaluate each model on the group that was omitted. For n cases, n-fold cross-validation would correspond to leave-one-out. But with a smaller k, we can perform the evaluation much more efficiently.
When doing cross-validation, there is the danger of affecting the error estimates with an arbitrary assignment to groups. In fact, one would wonder how does k-fold cross-validation compare to repeatedly splitting 1/k of the data into the hidden set and (k-1)/k of the data into the shown set.
As to compare cross-validation with random splitting, we did a small experiment, on a medical dataset with 286 cases. We built a logistic regression on the shown data and then computed the Brier score of a logistic regression model on the hidden data. The partitions were generated in two ways, using data splitting and using cross-validation. The image below shows that 10-fold cross-validation converges quite a bit faster to the same value as does repeated data splitting. Still, more than 20 replications of 10-fold cross-validation are needed for the Brier score estimate to become properly stabilized. Although it is hard to see, the resolution of the red line is 10 times lower than that of the green one, as cross-validation provides an assessment of the score after completing the 10 folds.
The implication is that the dependence of folds within a cross-validation experiment is a good thing if one tries to quickly assess the score. This dependence was seen as a problem before.
[Changes: the new picture does not show intermediate scores within each cross-validation, only the average score across all the cross-validation experiments.]
Boris Shor writes:
An article by EJ Dionne about our red-blue paper ("Rich state, poor state, red state, blue state: What's the matter with Connecticut?") appeared today in the Washington Post. Dionne had called me on Friday to talk about the paper, and we had a nice conversation -- he's a very sharp guy and really understood what we were trying to explain.
A couple of thoughts about the paper...
There is some exciting development happening in data mining. In particular, several tools are offering data-flow interfaces for data analysis. The core idea of a data-flow interface is to graphically depict the operations on data: for example, we load data from a data source (specifying the file name, types of variables, and so on). We can examine the data by passing it into a scatter-plot module. Alternatively, we can passes the data into an imputation module. From the imputation module we pass the data without missing values into a model testing module, along with the specifications of several statistical models (logistic regression, naive Bayes, SVM, and so on). Note that what flows in this case is the specification of the model, and not data or imputed data as before. The results of testing then flow into the ROC (receiver operating characteristic) module to be analyzed. I have done this in Orange, a freely available Python-based system for data analysis, and the whole program can be shown graphically:
The resulting diagram corresponds to a Python script, but it is easier to work with diagrams than with scripts, especially for beginning analysts. It is possible to connect Orange with R quite easily using the RPy module. I've been using it over the past few weeks with quite good results. It would be possible to create "widgets" for different R-based models.
Otherwise, one of the first tools with a data-flow interface was IBM's Visualization Data Explorer, but it was discontinued by IBM and transferred into open source OpenDX. The data mining kit Weka has a data-flow interface, but I prefer to use their Explorer. Finally, SPSS Clementine is a commercial data mining system with a data-flow interface.
Developing methodology for analyzing data is an interest of several communities. While there are many, I have wandered around statistics, machine learning and data mining. Every community has its own priorities, its own problems, its own preferred approaches. It is somewhat difficult for truly interdisciplinary work to emerge, but it is possible to examine what other communities are working on, and adopt good techniques from different areas. This posting will attempt to describe the motivations and interests of statisticians, machine learners and data miners.
There do not need to be many wanderers, but there are areas with relatively few. There was an interesting discussion in the blogosphere last year started by Eszter Hargittai and Cosma Shalizi about the clustering in the network of social networkers: physicists cite other physicists and sociologists cite other sociologists, without much intercitation. With the overproduction of scientific publications, researchers in the field of scientometrics like Loet Leydesdorff are trying to create maps of the scientific enterprise, using the citation links as indicators of connectedness. A good example is his Visualization of the Citation Impact Environments of Scientific Journals.
In the succeeding paragraphs, I will describe the different disciplines.
Christophe Andrieu gave a talk at IceBugs on adaptive MCMC for Bugs. I wasn't able to attend the meeting, but the presentation looks reasonable to me. Right now, one of my problems with Bugs is that sometimes it crashes--I think because it's using fancy stuff like slice sampling. I'd rather see it using the Metropolis algorithm, which never crashes.
The only thing about Andrieu's talk that I question is the emphasis on "vanishing adaptation"--that is, adaptation where the algorithm still converges to the correct target distribution. This is OK, I guess, but I think any adaptation is OK in the sense that it can eventually stop, and then you'll get the correct stationary distribution. After all, we need to do some burn-in anyway, so it makes sense to me to adapt for the first (burn-in) half and then simulate from there. (Then, if convergence hasn't been reached, one can use these last half of simulations to do another adaptation, and so forth.) So I don't know that any special theory is needed.
(Here's my own paper (with Cristian Pasarica) on the topic, where we have success adapting Metropolis jumps by optimizing the expected squared jumped distance. Our algorithm is great, but I have to admit it's pretty complicated, and Jouni and I have not gotten around to implementing it in Umacs.)
Following up on this, and in answer to the question in Section 1.5 of Andrieu's presentation, yes, adaptation can be done automatically. In fact, we do it in Umacs, adapting both the jumping scale and (for multivariate jumping rules) the shape of the jumping kernel.
P.S. Maybe it's the adaptive rejection sampling that's causing Bugs to crash--see Radford's comment.
Try the following in R:
y <- rcauchy (10000)hist (y)
It doesn't look like those pictures of a Cauchy distribution . . .
Jouni wrote a little R program that allows you to write a Bugs model as an R function. His "fitbugs" program then parses the R function and runs Bugs. It's just a convenience, but it allows you to set up the Bugs model and specify data, parameters, and initial values, all in one place. This is a little more convenient than having to put the Bugs model in a separate file.
Here's an example:
## Example: this is the bugs model written as an R function:
## Note that the data variables, the list of the variables to save, and
## the list of the variables to initialize are given as comments inside the function.
##
.example.bugs.model <- function () {
# data : n, J, y, state, income
# param : a, b, mu.a
# a = rnorm(J)
# b = rnorm(1)
# mu.a = rnorm(1)
# sigma.y = runif(1)
# sigma.a = runif(1)
#
for (i in 1:n) {
y[i] ~ dnorm(y.hat[i], tau.y)
y.hat[i] <- a[state[i]] + b*income[i]
}
b ~ dnorm(0, .0001)
tau.y <- pow(sigma.y, -2)
sigma.y ~ dunif (0, 100)
for (j in 1:J) {
a[j] ~ dnorm (mu.a, tau.a)
}
mu.a ~ dnorm(0, .0001)
tau.a <- pow(sigma.a, -2)
sigma.a ~ dunif (0, 100)
}
## Then, you'd call:
##
## fitbugs(.example.bugs.model)
##
Tim Besley sent me this paper by himself and Ian Preston on the relation between bias in an electoral system and the policies of political parties. They write, "The
results suggest that reducing electoral bias leads parties to moderate their policies."
Their model goes as follows: they start with a distribution of voter positions (in a one-diemensional ideology space) in each district, which, in combination with party issue positions, induces a two-party vote result in each district and thus a seats-votes curve. Properties of the seats-votes curve correspond to properties of the distribution of voter ideology distributions across districts.
They then run regressions on a dataset of local governments in Britain and find that, in the places with more electoral bias (thus, one party having a lot of "wasted votes" and the other party winning districts with just over 50% of the vote), the parties take on more extreme positions. The first way I would understand these results is geographically: areas with high bias must have the voters of one party or the other highly concentrated in districts. I'd like to know what these areas look like: are they inner cities favoring the Labour Party? Suburbs and rural areas favoring the Conservatives? I don't know enough about U.K. politics here, but I'd like to see a more detailed local analysis to complement the theoretical model. In particular, is it the districting, or is it just that, in the areas in which it is natural for districtings to be biased, the voters as a whole are more extreme? To start with, I'd think they could account for some of this by considering %vote for the parties as a predictor in their model.
Many scientists of the "selfish gene" persuasion get bothered by instances of altruistic behavior by humans and other animals. For example, Damon Centola forwarded these links:
Human beings routinely help others to achieve their goals, even when the helper receives no immediate benefit and the person helped is a stranger. Such altruistic behaviors (toward non-kin) are extremely rare evolutionarily, with some theorists even proposing that they are uniquely human. Here we show that human children as young as 18 months of age (prelinguistic or just-linguistic) quite readily help others to achieve their goals in a variety of different situations.
Evolutionary theory predicts that altruistic interactions, which are costly to the actor and beneficial to the recipient, will be limited to kin or reciprocating partners. This precludes anonymous acts of altruism on behalf of strangers, such as giving blood, or large-scale cooperation, such as serving on committees. . . . It’s not clear why chimpanzee infants were helpful to humans, but older chimpanzees did not help other chimpanzees obtain food rewards even when there was no cost in doing so.
I'm puzzled about the puzzlement
Caroline Korves writes,
We have dyadic data on sexual partnerships between individuals and want to analyze data at the partnership (dyad) level. The data come from a structured social network questionnaire. The outcome measure of interest is sexual concurrency practiced by at least one member of the dyad (so if one or both partners have concurrent partners then response =1, otherwise response=0). We want to look at what factors are associated with concurrency, since concurrency drives the spread of HIV and other STIs. As an example, one factor might be whether or not there is an age difference of at least five years between partners.
I appreciate all the comments here on my article on ANOVA for the New Palgrave Dictionary of Economics. The main suggestion was that I clarify what ANOVA gives you that regression doesn't. I hope this revision does the trick. Here's the revised article and here's the added section:
The analysis of variance is often understood by economists in relation to linear regression (e.g., Goldberger, 1964). From the perspective of linear (or generalized linear) models, we identify ANOVA with the structuring of coefficients into batches, with each batch corresponding to a ``source of variation'' (in ANOVA terminology).As discussed by Gelman (2005), the relevant inferences from ANOVA can be reproduced using regression---but not always least-squares regression. Multilevel models are needed for analyzing hierarchical data structures such as the split-plot design in Figure 2, where between-plot effects are compared to the main-plot errors, and within-plot effects are compared to sub-plot errors.
Given that we can already fit regression models, what do we gain by thinking about ANOVA? To start with, there are settings in which the sources of variation are of more interest than the individual effects. For example, the analysis of internet connect times in Figure 3 represents thousands of coefficients---but without taking the model too seriously, we can use the ANOVA display as a helpful exploratory summary of data. For another example, the two plots in Figure 4 allow us to quickly understand and compare two multilevel logistic regressions, again without getting overwhelmed with dozens of coefficient estimates.
More generally, we think of the analysis of variance as a way of understanding and structuring multilevel models---not as an alternative to regression but as a tool for summarizing complex high-dimensional inferences, as can be seen, for example, in Figure 5 (finite-population and superpopulation standard deviations) and Figures 6-7 (group-level coefficients and trends).
Also, I thanked the commenters who gave their full names. If anyone else has suggestions and also wants to be achknowledged in the article, just let me know your name. Thanks again.
Robin Varghese points to this paper by James Galbraith and Travis Hale, "State Income Inequality and Presidential Election Turnout and Outcomes." At a technical level, they offer a new state-level inequality measure that they claim is better than the measures usually used. I haven't read this part of the paper carefully enough to evaluate these claims. They then run some regressions of state election outcomes on state-level inequality, and find that "high state inequality is negatively correlated with turnout and positively correlated with the Democratic vote share, after controlling for race and other factors." So far, so good, I suppose (although I recommend that they talk with a statistician or quantitative political scientist about making their results more interpretable--I'd recommend they use the secret weapon, but at the very least they could do things like transform income into percentage of the national median, so they don't have coefficients like "4.58E-06").
Noooooooooo......
But then they write:
We [Galbraith and Hale] can, however, infer that the Democratic Party has engaged in campaigns that have resonated with both the elite rich and the comparatively poor.
Well, no, you can't infer that from aggregate results! To state it in two steps:
1. Just because state-level inequality is correlated with statewide vote for the Democrats, this does not imply that individual rich and poor voters are supporting the Democrats more than the Republicans. To make this claim is to make the ecological fallacy.
2. The Democrats do much better than the Republicans among poor voters, and much worse among the rich voters. (There's lots of poll data on this; for example, see here.) So, not only are Galbraith and Hale making a logically false inference, they are also reaching a false conclusion.
This example particularly bugs me because it's something my colleagues and I have worked on (see here, or here for a couple of pretty pictures).
To conclude on a more polite note
I don't want to be too hard on Galbraith on Hale. The essence of a "fallacy," after all, is not merely that it's a mistake, but that it's a tempting mistake--that it seems right at the time. (Otherwise we wouldn't have to warn people about these things.) Through the wonders of the www, the paper reached me, I noticed the mistake, I'll notify the authors, and they can fix this part of the paper and focus it more on the inequality measure itself, which is presumably where their paper started in the first place.
P.S. I certainly don't see my role in life as policing the web, looking for statistical errors. I just happened to notice this one, and the topic is something we've been researching for awhile. (And, of course, maybe I missed something important myself here...)
P.P.S. Galbraith and Hale have revised their paper to remove the implication about individual voters. I agree with them that this is an interesting topic to study--the key is, I think, to be able to work in the individual-level poll data to address questions of interest.
I'd like to plug my paper, Exploratory data analysis for complex models (with a friendly discussion by Andreas Buja (who originally solicited the paper for the journal) and a rejoinder by me).
I think this paper will be of interest to many of you because (a) people respond positively to statistical graphics, but (b) generally think of graphs as methods for displaying raw data.
In my experience, graphs of statistical inferences--estimates from models--can be extremely useful.
A Statistical Model of a Wireless Call
Diane Lambert, Google
A wireless call requires the cooperation of a dynamic set of base stations and antennas with control of the call changing in response to changes in signal strength. The communication between the mobile and the base station that is needed to manage the call generates a huge amount of data, some of which is seen only by the mobile placing the call and some of which is seen by the base station that manages the call. I [Lambert] will describe some of the subtleties in the data and a statistical model that takes the time dependent, spatial, and multivariate nature of the many wireless signals used to maintain a call into account. Online estimation and model validation using data taken from a commercial wireless network will also be discussed.
Joint work with David James and Chuanhai Liu (statistics) and A. Buvaneswari, John Graybeal and Mike McDonald (wireless engineering).
She'll be speaking in the statistics dept. seminar at Columbia next Monday at 4pm.
The second edition of "Intro Stats," by DeVeaux, Velleman, and Bock, is coming out. To request an examination copy, please contact your local Addison-Wesley representative (eugene.smith@aw.com). This (or its sister book, "Stats") is my favorite undergraduate introductory statistics book.
Building predictive models from data is a frequent pursuit of statisticians and even more frequent for machine learners and data miners. The main property of a predictive model is that we do not care much about what the model is like: we primarily care about the ability to predict the desired property of a case (instance). On the other hand, for most statistical applications of regression, the actual structure of the model is of primary interest.
Predictive models are not just an object of rigorous analysis. We have predictive models in our heads. For example, we may believe that we can distinguish good art from bad art. Mikhail Simkin has been entertaining the public with devious quizzes. A recent example is An artist or an ape?, where one has to classify a picture based on whether it was painted by an abstractionist or by an ape. Another quiz is Sokal & Bricmont or Lenin?, where you have to decide if a quote is from Fashionable Nonsense or from Lenin. There are also tests that check your ability to discern famous painters, authors and musicians from less famous ones. The primary message of the quizzes is that the boundaries between categories are often vague. If you are interested in how well test takers perform, Mikhail did an analysis of the True art or fake? quiz.
These quizzes bring us to another notion, which has rocked the machine learning community over the past decade: the notion of a support vector machine. The most visible originator of the methodology is Vladimir Vapnik. There are also close links to the methodology of Gaussian processes, and the work of Grace Wahba.
A SVM is nothing but a hyperplane in some space defined by the features. The hyperplane separates the cases of one class (ape pictures) from the cases of another class (painter pictures). Since there can be many hyperplanes that do separate one from the other, the optimal one is thought to be equidistant from the best ape picture and the worst painter picture. Using the `kernel trick' we can conjure another space where individual dimensions may correspond to interactions of features, polynomial terms, or even individual instances.
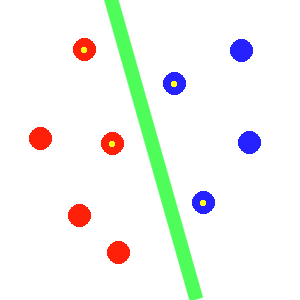
In the above image, we can see the separating green hyperplane halfway between the blue and red points. Some of the points are marked with yellow dots: those points are sufficient to define the position of the hyperplane. Also, they are the ones that constrain the position of the hyperplane. And this is the key idea of support vector machines: the model is not parameterized in terms of the weights assigned to features but in terms of weights associated with each case.
The heavily-weighted cases, the support vectors, are also interesting to look at, because of pure human curiosity. An objective of experimental design would be to do experiments that would result in new support vectors: otherwise the experiments would not be interesting - this flavor of experimental design is referred to as `active learning' in the machine learning community. The support vectors are the cases that seem the trickiest to predict. My guess is that Mikhail intentionally selects such cases in his quiz as to make it fun.
I was asked by the editors of the New Palgrave Dictionary of Economics (second edition) to contribute a short article on the analysis of variance. I don't really know what economists are looking for here (the article is supposed to be aimed at the level of first-year graduate students), but I gave it a try. Here's the article. Any comments (from economists or others) would be appreciated.
P.S. See here for revised version.
Today in the Collective Dynamics Seminar, Gueorgi will be discussing a set of papers on the distribution of weighting times for correspondence. The topic apparently is controversial. I can't quite see where the controversy lies, although it's clear from reading the language of the comments and replies that some people are getting angry. I'll first give the references, then my thoughts:
I saw a funny (and disturbing) movie last week, "Our Brand is Crisis", a documentary that follows some people from James Carville's political consulting firm as they advise a candidate in the 2002 presidential election campaign in Bolivia. The main consultant was a 40ish guy named Jeremy Rosner, who looked a lot like Ben Stiller and whos motto was "progressive politics and foreign policy for profit." (The Bolivian presidential candidate, "Goni," looked a bit like Bill Clinton.) The movie showed lots of strategy sessions (complete with ugly 3-d bar graphs) and focus groups (who sat around talking and eating potato chips; it was pretty funny). I was actually wondering how a Bolivian candidate could afford such expensive political consultants, but I suppose most of the cost was local labor (running the focus groups, polling, etc.) which must be pretty cheap.
I perhaps should be embarrassed to admit that I knew nothing about Bolivia before seeing the movie and thus was in suspense throughout. They said at some point in the movie that there were 11 candidates running, and I would have liked a little more background on the other 10 candidates to get a sense of where they stood and who was supporting them. I don't think I'm giving anything away when I say that the election itself was very close.
I was amazed at how much the consultants allowed Rachel Boynton (the director, no known relation to Sandra) to film all their private meetings. Some of the things they said were pretty embarrassing, I'd think--like the bit where they plan attack ads. But I guess it's all advertising for their firm.
There was a hilarious cameo by the U.S. Ambassador ("California will only buy your natural gas if Bolivia is not involved in cocaine").
At the end, there was a disturbing exchange where Boynton asks Rosner how he felt about what's been happening in Bolivia and he made some comment about the difficulty of establishing a "commitment to democracy" in poor countries. It was sort of weird because I didn't see any evidence that the Bolivians didn't want democracy, even if they were dissatisfied with particular political leaders.
Finally, one of the underlying concerns of everyone was Bolivia's economy, its budget crunch, and unemployment. "Jobs" was a key issue, and a lot of people felt that jobs were being sent overseas. The funny thing is, in the U.S. we wouldn't be so happy if we felt our jobs were being sent to Bolivia. So I wasn't quite sure how to think about this issue (international economics not being my area of expertise, to say the least).
Anyway, the movie was entertaining and thought-provoking, and I highly recommend it, expecially for anyone interested in polling and elections. For those of you in NYC, it'll be at the Film Forum starting Mar 1.
Sam Clark read my paper on game theory and trench warfare, and writes, "I agree that what really has to be explained is why soldiers shoot, not why they do not shoot. In particular, I am interested in how commands use military medals and decorations to get soldiers to risk their lives. Do you know of any work along this line?"
I don't actually know anthing more about this. Does anyone have anything useful on this (beyond the references in my paper)?
David from Alicia Carriquiry's class at Iowa State asks,
We have a question on Gelman's A Bayesian formulation of exploratory data analysis and goodness-of-fit testing". He writes, "Gelman, Meng, and Stern (1996) made a case that model checking warrants a further generalization of the Bayesian paradigm, and we continue that argument here. The basic idea is to expand from p(y|theta)p(theta) to p(y|theta)p(theta)p(y^rep), where y^rep is a replicated data set of the same size and shape as the observed data." (p. 5 of the PDF)Here and elsewhere he makes it sound like the distribution of y^rep is something that must be specified in addition to the prior and the likelihood... But don't the prior and the likelihood (plus the data at hand) force a unique choice for the distribution of y^rep? I thought this was how we defined the replication distribution in 544 (and it seems like they do the same in the 1996 paper. I've only skimmed that so far). y^rep follows the posterior predictive distribution.
Why does y^rep provide an extra "degree of freedom"/force modelers to do more thinking?
My reply: the choice in y^rep is what to condition on. For example, in a study with n data points, should y^rep be of length n, or should n itself be modeled (e.g., by a Poisson dist), so that you first simulate n^rep and then y^rep? Similar questions arise with hierarchical models: do you simulate new data from the same groups or from new groups?
This topic interests me because the directed graph of the model determines the options of what can be conditioned on. For example, we could replicate y, or replicate both theta and y, but it doesn't make sense to replicate theta conditional on the observed value of y. In Bayesian inference (or, as the CS people call it, "learning"), all that matters is the undirected graph. But in Bayesian data analysis, including model checking, the directed graph is relevant also. This comes up in Jouni's thesis.
Gregg Keller asks,
When setting up a regression model with no obvious hierarchical structure, with normal distribution priors for all the coefficients (where the normal distribution's mean and variance are also defined by a prior distribution), does it make sense to give the prior variance distributions for the various coefficients a shared component, or is it better to give each coefficient a completely independent set of prior distributions with no shared components?For instance, in Prof. Radford Neal's bayesian regression/neural network software, he gives all the inputs a common prior variance distribution (with an optional ARD prior to allow some variations). Is there any obvious reason to do this in a regression model?
My response: first off, I love Radford's book, although I haven't actually fit his models so can't comment on the details there. And to give another copout, it's hard to be specific without knowing the context of the problem. That said, when there are many predictors, I think it should be possible to structure them (see Section 5.2 of this paper or, for some real examples, some of Sander Greenland's papers on multilevel modeling). With many variance parameters, these themselves can be modeled hierarchically (see Section 6 of this paper for an exchangeable half-t model). I think that some of your questions about variance components can be addressed by a hierarchical model of this sort; the recent work of Jim Hodges and others on this topic is also relevant (actually, it's probably more relevant than my own papers).
Katherine Valdes sent us a link to this newspaper column by Richard Cohen, "Math courses don't add up." He begins with a sad story about a high-school student in L.A. who dropped out "after failing algebra six times in six semesters, trying it a seventh time and finally just despairing over ever getting it." He continues,
Sean Schubert pointed me to this article, " Figure Skating Scoring Found to Leave Too Much to Chance":
The overseers of international figure skating scoring instituted a new system in 2004, designed to reduce the chances of vote fixing or undue bias after the scandal during the Winter Olympics in Salt Lake City in 2002. Under the old rules eight known national judges scored a program up to six points with the highest and lowest scores dropped. Under the new rules, 12 anonymous judges score a program on a 10-point scale. A computer then randomly selects nine of the 12 judges to contribute to the final score. The highest and lowest individual scores in each of the five judging categories are then dropped and the remaining scores averaged and totaled to produce the final result.This random elimination of three judges results in 220 possible combinations of nine-judge panels, explains John Emerson, a statistician at Yale University. And according to his analysis of results from the shorts program at the Ladies' 2006 European Figure Skating Championships, the computer's choice of random judges can have a tremendous--and hardly fair--impact on the skaters' rankings. "Only 50 of the 220 possible panels would have resulted in the same ranking of the skaters following the short program," Emerson writes in a statement announcing his findings.
I have to say, selecting judgments at random seems pretty wacky to me. Why not just average all of them? I also think it's funny that the ratings are from 0 to 10, with increments of 0.25. Why not just score them from 0 to 40 with increments of 1, or 0 to 4 with increments of 0.1?
The article does point out an interesting problem, which is that judges are perhaps giving too-low scores at the beginning to leave room later. The system of adding or deducting points from the base value seems like a step toward fixing this. But they should set the base value low (e.g., at 3, rather than at 7), so that they'll have more resolution at the upper end of the scale. As things stand, the scale might be better designed for picking the worst skater than the best!
On the other hand, I'm not so strongly moved by Emerson's argument that removing different judges would change the outcome. As Tom Louis has written, rankings are pretty random anyway, and, in any case, things would be different if a different set of 12 judges were selected.
Chad d'Entremont writes,
Eric Archer forwarded this document by Nick Freemantle, "The Reverend Bayes--was he really a prophet?", in the Journal of the Royal Society of Medicine:
Does [Bayes's] contribution merit the enthusiasms of his followers? Or is his legacy overhyped? . . .First, Bayesians appear to have an absolute right to disapprove of any conventional approach in statistics without offering a workable alternative—for example, a colleague recently stated at a meeting that ‘. . . it is OK to have multiple comparisons because Bayesians’ don’t believe in alpha spending’. . . .
Second, Bayesians appear to build an army of straw men—everything it seems is different and better from a
Bayesian perspective, although many of the concepts seem remarkably familiar. For example, a very well known
Bayesian statistician recently surprised the audience with his discovery of the P value as a useful Bayesian statistic at a meeting in Birmingham.Third, Bayesians possess enormous enthusiasm for the Gibbs sampler—a form of statistical analysis which
simulates distributions based on the data rather than solving them directly through numerical simulation, which they
declare to be inherently Bayesian—requiring starting values (priors) and providing posterior distributions (updated
priors). However, rather than being of universal application, the Gibbs sampler is really only advantageous in a
limited number of situations for complex nonlinear mixed models—and even in those circumstances it frequently
sadly just does not work (being capable of producing quite impossible results, or none at all, with depressing
regularity). . . .
The looks negative, but it you read it carefully, it's an extremely pro-Bayesian article! The key phrase is "complex nonlinear mixed models." Not too long ago, anti-Bayesians used to say that Bayesian inference was worthless because it only worked on simple linear models. Now their last resort is to say that it only works for complex nonlinear models!
OK, it's a deal. I'll let the non-Bayesians use their methods for linear regression (as long as there aren't too many predictors; then you need a "complex mixed model"), and the Bayesians can handle everything complex, nonlinear, and mixed. Actually, I think that's about right. For many simple problems, the Bayesian and classical methods give similar answers. But when things start to get complex and nonlinear, it's simpler to go Bayesian.
(As a minor point: the starting distribution for the Gibbs sampler is not the same as the prior distribution, and also that Freemantle appears to be conflating a computational tool with an approach to inference. No big deal--statistical computation does not seem to be his area of expertise--it's just funny that he didn't run it by an expert before submitting to the journal.)
Also, I'm wondering about this "absolute right to disapprove" business. Perhaps Bayesians could file their applications for disapproval through some sort of institutional review board? Maybe someone in the medical school could tell us when we're allowed to disapprove and when we can't.
P.S.
Yes, yes, I see that the article is satirical. But, in all seriousness, I do think it's a step forward that Bayesian methods are associated with "complex nonlinear mixed models." That's not a bad association to have, since I think complex models are more realistic. To go back to the medical context, complex models can allow treatments to have different effects in different subpopulations, and can help control for imbalance in observational studies.
Naseem Taleb's publisher sent me a copy of "Fooled by randomness: the hidden role of chance in life and the markets" to review. It's an important topic, and the book is written in a charming style--I'll try to respond in kind, with some miscellaneous comments.
I saw David Stork give yesterday the best talk I've ever seen. Here's the abstract:
In 2001 artist David Hockney and scientist Charles Falco stunned the art world with a controversial theory that, if correct, would profoundly alter our view of the development of image making. They claimed that as early as 1420, Renaissance artists employed optical devices such as concave mirrors to project images onto their canvases, which they then traced or painted over. In this way, the theory attempts to explain the newfound heightened naturalism of painters such as Jan van Eyck, Robert Campin, Hans Holbein the Younger, and others. This talk for general audiences, lavishly illustrated with Renaissance paintings, will present the results of the first independent examinations of the projection theory. The analyses rely on sophisticated computer vision and image analysis algorithms as well as computer graphics reconstructions of Renaissance studios. While there remain some loose ends, such rigorous technical analysis of the paintings, infra-red reflectograms, modern reenactments, internal consistency of the theory, and alternate explanations allows us to judge with high confidence the plausibility of this bold theory. You may never see Renaissance paintings the same way again.
P.S. Commenter John S. points out this fascinating website on the topic. Here's a summary of Stork's findings.
The evaluation of decision trees under uncertainty is difficult because of the required nested operations of maximizing and averaging. Pure maximizing (for deterministic decision trees) or pure averaging (for probability trees) are both relatively simple because the maximum of a maximum is a maximum, and the average of an average is an average. But when the two operators are mixed, no simplification is possible, and one must evaluate the maximization and averaging operations in a nested fashion, following the structure of the tree. Nested evaluation requires large sample sizes (for data collection) or long computation times (for simulations).
An alternative to full nested evaluation is to perform a random sample of evaluations and use statistical methods to perform inference about the entire tree. We show that the most natural estimate is biased and consider two alternatives: the parametric bootstrap, and hierarchical Bayes inference. We explore the properties of these inferences through a simulation study.
Here's the paper (by Erwann Rogard, Hao Lu, and myself).
Richard asks if the patterns in our red/blue paper can be explained by different income distributions in different states. In particular, the income quantiles we use (which come from the National Election Study, and which we use so we can have comparable estimates across states and across years) are based on national income quantiles. Thus, the people in the highest income category in Mississippi are richer (compared to average Mississippians) than the people in the highest income category in Connecticut (compared to average Connecticutians). Similarly, the people in the lowest income category in Mississippi are richer (compared to average Mississippians) than the people in the highest income category in Connecticut (compared to average Connecticutians).
We were certainly aware of this in writing the paper (in fact, the sizes of the circles in our graph make clear that the relative proportions of each of the categories varies from state to state). The question is: can this explain away our results? As Richard writes,
So here's an alternative hypothesis for the results they're getting: in each income quantile the Mississippians feel richer than the Connecticutters in the same quantile and therefore they vote Republican. This can be seen as a slight twist on the economic determinism argument, with subjective, relative income replacing objective, absolute income as the determinant.So would correcting for this make the effect they found go away? Probably not... or at least it's not clear that it would. But it would be interesting to see how the model held up on state-specific quantiles and/or COL adjusted ones. Would the top 2% of Connecticutters be more Republican than the top 8%, thus pushing the slope positive and diminishing the overall effect?
My response: this could explain some of the difference in intercepts of the regression lines, but I don't see it explaining the differences in the slopes, which is what we're most interested in.
However, it might be a worthwhile calculation to set up a model (e.g., a logistic regression of preference on individual income relative to state-average income) and see how much things change by switching to national-average income as a baseline. Due to discreteness in the data, it would be tough to do this by simply running a new regression, but with a little modeling it shouldn't be hard to estimate the size of this scaling effect.
From Michael Stastny comes a link to two articles with advice and more advice from Peter Kennedy on the practicalities of econometrics (what we in statistics would call "regression analysis," I think). As a writer of textbooks and also a consumer of such methods in my applied work, I'm interested in this sort of general advice.
While on the web looking for something else (of course), I discovered this "Ask E.T." page where graphics expert Ed Tufte answers questions dealing with information design. Lots of interesting comments on these threads by Howard Wainer and others.
The only thing I find mysterious is some of the threads are labeled with three asterisks. And near the top of the page, we see this:
*** = 3-star threads
Yeah, sure, but what does that mean? Funny to see this kind of content-less note on a Tufte site, of all places.
There have been several news stories about a recently completed study of the effects of a low-fat diet on heart disease, colon cancer, and breast cancer. One of those stories is here. Some excerpts:
The large primary prevention trial conducted at 40 U.S. clinical centers from 1993 to 2005 enlisted 48,835 postmenopausal women ages 50 to 79 years.
Women in all three trials were randomly assigned to an intervention group (40%, n=19,541) with intensive behavior modification sessions and the goal of reducing fat intake to 20% of energy and increasing vegetable and fruit intake to at least five servings a day and grains to six servings.
Participants in a comparison group (60%, n= 29,294) were not asked to make changes...
So far, so good: we know the sample sizes, we know something about the interventions. What about the results? The article goes on to say:
Over an 8.1-year average follow-up, a low-fat diet did not lead to a significant reduction in invasive breast cancer risk, the researchers reported.
The number of women (annualized incidence rate) who developed breast cancer was 655 (0.42%) in the intervention group and 1,072 (0.45%) in the comparison group (hazard ratio 0.91; 95% confidence interval 0.83-1.01).
For starters, 655 cases out of 19541 women in the intervention group is 3.3%, not 0.42%, so I'm not sure quite what is going on with the numbers. Presumably something got lost in translation from the scientific publication to the news article.
But the thing that really strikes me is that the 95% confidence interval for breast cancer prevention just barely includes 1.00, or no effect. So the news stories all say some variation of "there is no significant benefit" from a low-fat diet. I suspect, though of course we will never know for sure, that if there had been just a couple fewer cases in the intervention group, or a couple more in the comparison group, so that the 95% confidence interval topped out at 0.99 rather than 1.01, the news articles would have trumpeted "Significant reduction in breast cancer from a low-fat diet." This is an issue Andrew touched on a few weeks ago on this blog.
Like a lot of people who perform statistical analyses, I've always been unhappy with "statistical significance" (the term, and the concept) for two reasons: (1) in common speech, "significant" means "important", but that is not true in stat-speak, where a parameter value can be "statistically significant" even though it is of no practical importance, and can be of great practical importance even if it is not "statistically significant" (the latter being the case if sample sizes or other sources of uncertainty are so large that even important effects can be missed); and (2) when did God say that 95% is the threshold for "statistical significance"? What's so magic about 1/20, as opposed to 1/18 or 1/21 or anything else for that matter?
In the current study, there is mild evidence for a small effect on breast cancer; the best guess (from this study alone) would suggest that a low-fat diet, of the type they tested, reduces breast cancer incidence by something between 5% and 15%, although larger or smaller benefits (or even a small penalty) cannot be ruled out. I wish reporters would put it that way, rather than declaring that the result did or did not meet some arbitrary (though admittedly customary) "significance" standard.
Jose Pedro Gala sends in some quesetions about statistical methods for causal inferences. I'll give his questions, then my responses. Jose writes:
Phil Henshaw posted a comment (via Aleks) on my entry on redistricting. I'll repeat Henshaw's comments, followed by my reactions.
Phil Henshaw writes,
Step 1: Speak at Duke.
Step 2: Make sure that Brendan Nyhan is in the audience.
Step 3: Make sure that Kevin Drum reads Brendan Nyhan and cites your article.
Step 4: Make sure that John Kastellec reads Kevin Drum and points you to this post with its 84 comments (so far).
I only wish that Kevin Drum would've linked to this pretty picture which I tihnk tells the story so cleanly:
and to our blog entries here and here on the topic.
Anyway, it's nice to get these ideas out there. To end on a technical note: Drum gives us a bit too much credit when he writes,
The chart above [Figure 13 of our paper] shows how clear this distinction is, and the authors report that it's become clearer over time. It's rare to get such a clean regression line in social science data like this, which makes their results pretty provocative.
It's not quite so clean as all that, however: the points on that graph are estimates from the multilevel model, not raw data, and the model itself pulls the points toward a straight line (to the extent that this line is supported by the data). See Footnote 9 on page 19 for an elaboration of this point. Just to be clear: I do believe this pattern is striking--and, amazingly enough, we discovered it in our analysis, we did not go looking for it. But the super-crispness of the line is somewhat a product of the modeling.
Delivery guy talking to secretary in the medical office: "Seattle had a nice team but Pittsbugh was hungrier. That's why they won."
The sad thing is, probably many of our students would say the same thing, even after our attempted brainwashing of them in Stat 1111.
P.S. See chapter 1 of our book for some football data (courtesy of Hal Stern). The point differential has a distribution that is centered at the point spread with an sd of 14 points.
Sudipto Banerjee and I had a little exchange, inspired by a comment on the Bugs mailing list, which might be of interest to the hierarchical modelers out there. It started with Wayne Throgmartin with a set of questions about fitting, understanding, and checking complex multilevel models.
Jim Snyder is speaking Fri 17 Feb at NYU on "Issue preferences and measurement error," his paper with Steve Ansolabehere and Jonathan Rodden. I think this is an important paper. First I'll give the abstract (again, here's the paper) and then my comments.
Ansolabehere, Rodden, and Snyder write:
We show that averaging a large number of survey items on the same broadly-defined issue area--e.g., government involvement in the economy, or moral issues--eliminates a large amount of measurement error and reveals issue preferences that are well structured. Averaging produces issues scales that are stable over time, and with enough items, these scales are often as stable as party identification. The scales also exhibit within-survey stability when we construct scales made from disjoint subsets of survey items. Moreover, this intertemporal and within-survey stability increases steadily, and in a manner consistent with a standard common measurement error model, as the number of survey items used increases. Also, when we estimate Converse's "black-white" model, we find that at most 20-25 percent of respondents can be classified as "pure guessers," and 75-80 percent have stable attitudes over issue areas, the reverse of Converse's conclusion. Finally, in regressions predicting presidential vote choice, the issue scales appear to have much more explanatory power--relatively large coefficients and much larger t-values--than any of the individual survey items used in constructing the scales.
My comments:
1. I think this paper is important for substantive reasons (it's interesting that voters are more issue-consistent than researchers have thought), but also statistically--iIm always trying to make the point to students and collaborators that it's a good idea to combine questions into "scores," and it's nice to have this example. Sometimes items can be combined using factor analysis, but often simple averages are effective (as Robyn Dawes and others have pointed out in the context of psychological measurement).
2. I wonder if they chould talk more about what is meant by "measurement error." This could be unstable preferences on individual items (e.g., a weak preference that is easily influenced by what was in the newspaper last week), or people not paying attention and answering randomly, or people not understanding the question, or different people having different ideas of what the question is, ...
The term "measurement error" could be misleading--it assumes a lot, in that it implicitly assumes that there is a "true response" that is "measured" with "error." I don't think the paper stands or falls on this assumption, so it might be good to detach it a bit from the main results.
3. Just to clarify my above comment: I think Section 2 of the paper is fine at a technical level but perhaps still leaves too much assumed about where the errors come from.
4. When referring to the black/white model, it would be good to refer also to some more sophisticated versions, for example the paper that Jennifer Hill and others wrote on this a few years back.
And some statistical comments:
Gueorgi writes,
I read in your blog a few days ago about "square" and "diamond" treated as different "shapes" in Curious George's Big Book of Curiosities.You may find it interesting that children of an indigenous Amazonian tribe are able to spontaneously ("in the absence of schooling") make use of rotation invariance and other geometric principles, according to this paper just published in Science:
The Statistics Research group in AT&T Labs invites applications for full time research positions. Applicants should have a Ph.D. in Statistics (or a related field) and be able to make major, widely-recognized contributions to statistics research: theory, methods, computing, and data analysis.
Job at Harris Interactive. Here's the link, and here's the description:
Seth told me once about a nutrition researcher, Dr. Ranjit Kumar Chandra, whose work Seth and a colleague read about a few years ago and realized was fradulent. There was some controversy at the time, but in retrospect the pattern of fraud was clear. Here's the transcript of a recent Canadian Broadcasting Company broadcast on the case (the doctor was based at a hospital in Newfoundland). Here's the basic story (from the broadcast):
Dsquared's comment on this entry mentioned the economist Deirdre McCloskey, whom I googled and found this paraphrase of a quote from Don Boudreaux, "that no one was ever convinced by raw data of the truth of a proposition that he or she did not already hold to be true."
I wonder what the original form of the quotation was. Or maybe what I really wonder is, what is the point of demarcation for which Boudreaux's statement is true? As written it is certainly false. I know this because I myself get convinced by raw data of propositions on which I held no prior opinion. Here are just a few recent examples:
- Rich people and poor people differ more in their political preferences in poor states than in rich states
- The NYC police department stopped more minorities than whites, even after controlling for neighborhoods and previous crime rates
- Americans have, on average, about 700 acquaintances each
- and many, many others.
And, as a bonus, here's an example where an analysis of raw data left me unconvinced of a hypothesis (that a local election was rigged).
I certainly did not "already hold" these propositions to be true--or false. In fact, in many cases (for example, the rich and poor states), the interesting proposition didn't even come to me until the data whacked me on the head with it.
Different sorts of propositions?
How, then, could Boudreaux (and McCloskey?) say such a thing? There must be different sorts of propositions. The propositions that I study tend to be technical--even the studies of voting and police stops are emprical questions, not value judgments. But for the big social questions, maybe it's hard for people to be persuaded by data because they already have such strong opinions. One thing I like about emprical science (including social science) is that I can be "convinced by raw data of the truth of a proposition that he or she did not already hold to be true"--and it happens all the time.
It's great to know that Shakespeare scholars are reading this blog. Alan Farmer (Department of English, Ohio State University) wrote to me several months ago:
I've recently co-authored an article that relies on statistical analyses of the early modern English book trade (specifically, the publication of plays from 1576 to 1660), and that article has elicited a response from someone in my field. In our rebuttal to that response, we want to point out that the chi square test addresses one of the respondent's central concerns and proves our point with a high degree of confidence, and we need to do so for an audience of literary critics (I believe this will be the first time the phrase "chi square test" has ever appeared in "Shakespeare Quarterly").
Seth forwarded me an article from the Chicago Tribune on mercury poisoning in fish. Near the end of the article, there's a mini-debate about composite sampling, which brought back some memories, since I did a project in 1994 on composite sampling of fish.
I'll post the relevant bits from my article and then give my comments.
Recent Comments